Table of Contents
Introduction
Generative AI also known as Generative Artificial Intelligence has rapidly emerged as a revolutionary technology capable of creating new content that closely mimics human creativity. This innovative branch of AI encompasses systems designed to generate text, images, music, and even video content, transforming the landscape of digital creativity and automation. The foundation of generative AI lies in sophisticated machine learning models trained on vast datasets to understand intricate patterns and produce novel outputs. As this technology evolves, it unlocks new possibilities while posing significant ethical and societal challenges.
Types of Generative AI Models
Generative AI primarily relies on three types of models: Generative Adversarial Networks (GANs), Variational Autoencoders (VAEs), and Transformer Models. Each of these models plays a crucial role in advancing the capabilities of generative AI.
- Generative Adversarial Networks (GANs): GANs consist of two neural networks, the generator and the discriminator, that work in tandem to produce increasingly realistic outputs. The generator creates new data instances, while the discriminator evaluates them against real data. Through this adversarial process, GANs generate remarkably authentic images, videos, and other forms of content.
- Variational Autoencoders (VAEs): VAEs encode data into a latent space and then decode it back, allowing the generation of new data similar to the original input. VAEs are particularly useful for generating images and other structured data, offering a more controlled and interpretable generation process than GANs.
- Transformer Models: Transformer models, such as the Generative Pre-trained Transformer (GPT), are primarily used for natural language processing tasks. These models can generate human-like text based on a given input, enabling applications ranging from essay writing to conversational agents. GPT-3, for instance, has demonstrated remarkable proficiency in generating coherent and contextually relevant text.
Applications of Generative AI
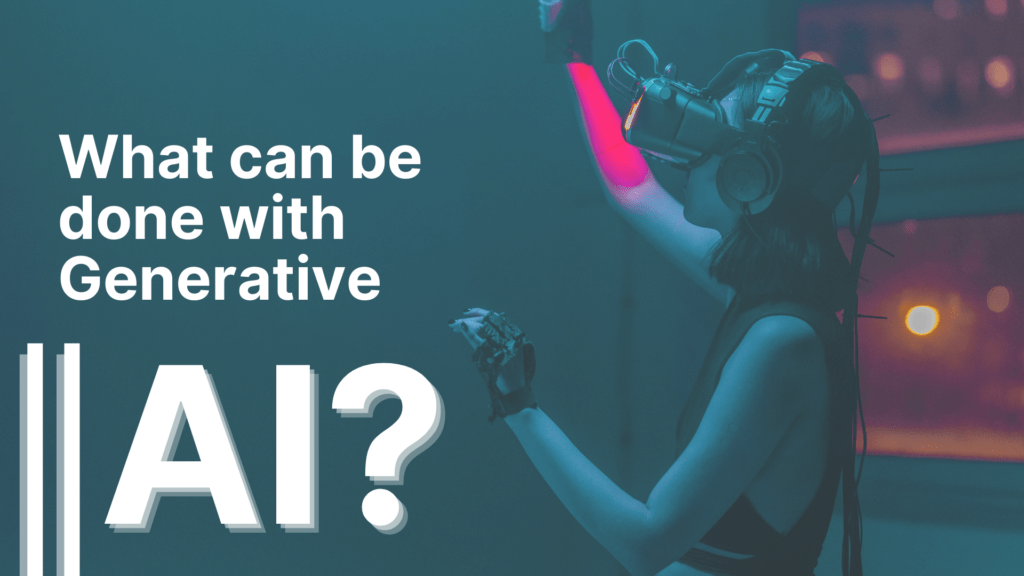
The applications of generative AI span across various domains, showcasing its versatility and transformative potential.
- Text Generation: Generative models like GPT-3 can write essays, articles, poetry, and code snippets. These models assist in automating content creation, enhancing productivity, and providing creative inspiration for writers and developers.
- Image Generation: GANs are widely used to create realistic images, which find applications in art, fashion, and advertising. Additionally, generative AI can generate synthetic data to train other AI models, addressing data scarcity issues in certain fields.
- Music and Audio: AI can compose original music pieces or generate realistic human speech. These capabilities are leveraged in entertainment, virtual assistants, and accessibility solutions, enriching the auditory experience for users.
- Video and Animation: Generative AI can create content, animate characters, and even produce deepfake videos. While these applications offer innovative possibilities in filmmaking and gaming, they also raise concerns about authenticity and ethical use.
Ethical Considerations
The rise of generative AI brings forth several ethical considerations that must be addressed to ensure responsible and beneficial use.
- Misinformation: The ability of generative AI to produce highly realistic fake news, deepfakes, and misleading information poses a significant threat to public trust and societal stability. Safeguards and regulatory measures are necessary to mitigate these risks.
- Copyright Issues: AI-generated content can potentially infringe on the intellectual property rights of original creators. Establishing clear guidelines and legal frameworks is crucial to protect both AI-generated and human-created content.
- Bias and Fairness: Generative models can perpetuate or amplify biases present in the training data, leading to discriminatory outputs. Ensuring diversity and fairness in training datasets and implementing bias mitigation techniques are essential to address this challenge.
Future Directions
The future of generative AI holds exciting possibilities and advancements that will shape various industries and societal dynamics.
- Improved Realism: Ongoing research aims to enhance the quality and realism of generated content, making it indistinguishable from human-created content. This progress will drive the adoption of generative AI in creative industries and beyond.
- Interactivity: Future generative models will become more interactive and responsive to user inputs, enabling dynamic and personalized content generation. This interactivity will revolutionize user experiences in applications such as virtual assistants and interactive storytelling.
- Integration: Combining generative AI with other emerging technologies, such as augmented reality (AR) and virtual reality (VR), will unlock new dimensions of immersive and interactive experiences. These integrations will redefine entertainment, education, and communication.
Conclusion
Generative AI represents a remarkable convergence of technology and creativity, offering unprecedented opportunities for innovation across diverse fields. Its ability to generate human-like content opens new frontiers in automation, entertainment, and data generation. However, the ethical implications and potential risks associated with generative AI must be carefully managed to ensure its responsible and beneficial use. As we continue to explore and develop this transformative technology, it is essential to strike a balance between innovation and ethical considerations, paving the way for a future where generative AI enriches our lives while upholding the values of fairness, authenticity, and trust.